一、环境与工程
参考上一节:Swin Transformer做主干的 Faster RCNN 目标检测网络
使用的是同一个工程,环境无需再次配置。
二、Swin Transformer YOLO3 网络代码
这里就没在分开写每一个部分了,mmdetection项目里面也是直接在一个文件里面全部写完的,应该是可复用的代码少吧。
1. 在configs/swin 目录下新建文件:yolov3_swin_mstrain-608_3x_coco.py
文件代码内容如下:
注意:
- img_scale 默认是 448*448,这个可以根据自己的显存大小适当调大、调小。最好是224的整数倍。
- 数据集配置部分参考B站教程:数据集标注2. 修改mmdet/datasets/ 下 coco.py
1
2
3
4
5
6
7
8
9
10
11
12
13
14
15
16
17
18
19
20
21
22
23
24
25
26
27
28
29
30
31
32
33
34
35
36
37
38
39
40
41
42
43
44
45
46
47
48
49
50
51
52
53
54
55
56
57
58
59
60
61
62
63
64
65
66
67
68
69
70
71
72
73
74
75
76
77
78
79
80
81
82
83
84
85
86
87
88
89
90
91
92
93
94
95
96
97
98
99
100
101
102
103
104
105
106
107
108
109
110
111
112
113
114
115
116
117
118
119
120
121
122
123
124
125
126
127
128
129
130
131
132
133
134
135
136
137
138
139
140
141
142
143
144
145
146
147
148
149
150
151
152_base_ = '../_base_/default_runtime.py'
pretrained = 'https://github.com/SwinTransformer/storage/releases/download/v1.0.0/swin_tiny_patch4_window7_224.pth'
# model settings
model = dict(
type='YOLOV3',
backbone=dict(
type='SwinTransformer',
embed_dims=96,
depths=[2, 2, 6, 2],
num_heads=[3, 6, 12, 24],
window_size=7,
mlp_ratio=4,
qkv_bias=True,
qk_scale=None,
drop_rate=0.,
attn_drop_rate=0.,
drop_path_rate=0.2,
patch_norm=True,
out_indices=(3, 2, 1),
with_cp=False,
convert_weights=True,
init_cfg=dict(type='Pretrained', checkpoint=pretrained)),
neck=dict(
type='YOLOV3Neck',
num_scales=3,
in_channels=[768, 384, 192],
out_channels=[512, 256, 128]),
bbox_head=dict(
type='YOLOV3Head',
num_classes=4,
in_channels=[512, 256, 128],
out_channels=[1024, 512, 256],
anchor_generator=dict(
type='YOLOAnchorGenerator',
base_sizes=[[(116, 90), (156, 198), (373, 326)],
[(30, 61), (62, 45), (59, 119)],
[(10, 13), (16, 30), (33, 23)]],
strides=[32, 16, 8]),
bbox_coder=dict(type='YOLOBBoxCoder'),
featmap_strides=[32, 16, 8],
loss_cls=dict(
type='CrossEntropyLoss',
use_sigmoid=True,
loss_weight=1.0,
reduction='sum'),
loss_conf=dict(
type='CrossEntropyLoss',
use_sigmoid=True,
loss_weight=1.0,
reduction='sum'),
loss_xy=dict(
type='CrossEntropyLoss',
use_sigmoid=True,
loss_weight=2.0,
reduction='sum'),
loss_wh=dict(type='MSELoss', loss_weight=2.0, reduction='sum')),
# training and testing settings
train_cfg=dict(
assigner=dict(
type='GridAssigner',
pos_iou_thr=0.5,
neg_iou_thr=0.5,
min_pos_iou=0)),
test_cfg=dict(
nms_pre=1000,
min_bbox_size=0,
score_thr=0.05,
conf_thr=0.005,
nms=dict(type='nms', iou_threshold=0.45),
max_per_img=100))
# dataset settings
dataset_type = 'CocoDataset'
data_root = 'data/coco/'
img_norm_cfg = dict(mean=[0, 0, 0], std=[255., 255., 255.], to_rgb=True)
train_pipeline = [
dict(type='LoadImageFromFile', to_float32=True),
dict(type='LoadAnnotations', with_bbox=True),
dict(
type='Expand',
mean=img_norm_cfg['mean'],
to_rgb=img_norm_cfg['to_rgb'],
ratio_range=(1, 2)),
dict(
type='MinIoURandomCrop',
min_ious=(0.4, 0.5, 0.6, 0.7, 0.8, 0.9),
min_crop_size=0.3),
dict(type='Resize', img_scale=[(448, 448)], keep_ratio=True),
dict(type='RandomFlip', flip_ratio=0.5),
dict(type='PhotoMetricDistortion'),
dict(type='Normalize', **img_norm_cfg),
dict(type='Pad', size_divisor=32),
dict(type='DefaultFormatBundle'),
dict(type='Collect', keys=['img', 'gt_bboxes', 'gt_labels'])
]
test_pipeline = [
dict(type='LoadImageFromFile'),
dict(
type='MultiScaleFlipAug',
img_scale=(448, 448),
flip=False,
transforms=[
dict(type='Resize', keep_ratio=True),
dict(type='RandomFlip'),
dict(type='Normalize', **img_norm_cfg),
dict(type='Pad', size_divisor=32),
dict(type='ImageToTensor', keys=['img']),
dict(type='Collect', keys=['img'])
])
]
data = dict(
samples_per_gpu=8,
workers_per_gpu=6,
train=dict(
type=dataset_type,
ann_file=data_root + 'annotations/instances_train2017.json',
img_prefix=data_root + 'train2017/',
pipeline=train_pipeline),
val=dict(
type=dataset_type,
ann_file=data_root + 'annotations/instances_val2017.json',
img_prefix=data_root + 'val2017/',
pipeline=test_pipeline),
test=dict(
type=dataset_type,
ann_file=data_root + 'annotations/instances_test2017.json',
img_prefix=data_root + 'test2017/',
pipeline=test_pipeline))
optimizer = dict(
type='AdamW',
lr=0.0001,
betas=(0.9, 0.999),
weight_decay=0.05,
paramwise_cfg=dict(
custom_keys={
'absolute_pos_embed': dict(decay_mult=0.),
'relative_position_bias_table': dict(decay_mult=0.),
'norm': dict(decay_mult=0.)
}))
optimizer_config = dict(grad_clip=None)
lr_config = dict(
policy='step',
warmup='linear',
warmup_iters=1000,
step=[27, 33])
runner = dict(type='EpochBasedRunner', max_epochs=36)
evaluation = dict(interval=1, metric=['bbox'])
CLASSES中填写自己的分类:例如CLASSES = ('person', 'bicycle', 'car')
。
当只有一个类别时,多加一个逗号:CLASSES = ('person',)
三、数据集
数据集依然使用默认的coco格式,数据集制作参考数据集标注(LabelImg、LabelMe使用方法)
四、训练模型
直接执行: python tools/train.py configs/swin/yolov3_swin_mstrain-608_3x_coco.py
注意:第一次执行会下载权值文件,需要等待一段时间,或者用特殊办法快点下载,权值文件会自动保存到你的电脑上,下次运行的时候就不再需要重新下载了,当然也可以和之前一样,提前下载好权值文件,然后配置一下。
五、测试训练效果
添加一个自己的图片在demo目录下,
执行:python demo/image_demo.py demo/000071.jpg configs/swin/yolov3_swin_mstrain-608_3x_coco.py work_dirs/yolov3_swin_mstrain-608_3x_coco/latest.pth
latest.pth 就是自己训练好的最新的权重文件,默认会放在workdir下。
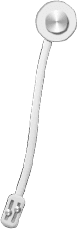
...
...
00:00
00:00
本文为作者原创文章,未经作者允许不得转载。